It seems obvious that despite 3 or 4 decades of research on Machine Learning, the ability of computers to learn is far inferior to that of humans.As a result it seems natural to choose as a goal for learning algorithms to match human performance.
However, is it really fair to compare computer learning with human learning? Or, more precisely, how should they be compared in order to have an objective assessment of their respective abilities?
Here are some remarks that one should have in mind before making such a comparison:
First of all, it is well known that, theoretically speaking, there is no better algorithm: if you look at all the possible problems, and compare any two algorithms, there will be exactly one half of the problems on which the first algorithm will do a better job than the second one. In other terms, there is no algorithm that systematically outperforms the other algorithms on all problems. It is thus pointless to compare learning algorithms in general.So in order to make a meaningful comparison, one should first select a limited number of problems of interest. The natural class of problems in our case would be to consider all the learning problems that humans are coping with: e.g. speech recognition, object recognition, motor learning...Having a list of such problems would make a reasonable benchmark on which learning algorithms could be evaluated and compared to human performance.
Of course, on all these tasks the algorithms we have built so far are unable to match (or in certain cases even get close to) human performance.Does it mean our algorithms are bad?I would tend to think rather the opposite: they are very good and much better than humans in many respects. In my opinion, the reason why they do not get good success rates on those problems that are trivially solved by humans is that they don't have access to the same information and they are not given the right "priors"
Human brains are genetically designed for solving certain types of learning problems. For example, there are plenty of hard wiring in the visual system that makes object recognition easy for humans.Also, for most "human learning" tasks, when we build a database of examples to be given to a computer, we only give a very limited amount of information to the computer. For example, when humans start to learn to recognize hand written characters, they already have a visual system that has been extensively trained to recognize all sorts of shapes. Imagine someone who has been blind from birth who suddenly recovers sight at the age of 6 and is directly put in front of handwritten characters on a screen, and asked to classify them. I would suspect that he would not get a better accuracy than existing learning algorithms.
A similar but more realistic example could be constructed as follows. Imagine you are presented with images of handwritten characters whose pixels have been shuffled in a deterministic way. For vector-based learning algorithms this would not make any difference (they would still get some good predictive accuracy), while for humans it would be completely impossible to reach any reasonable level of generalization.
Yet another example to illustrate the kind of task that computers are faced with is the spam classification. It is clear that humans are better at classifying spam vs non-spam emails. The reason is that they "understand" what the emails mean and this is because of years of language training. So now, imagine you are given 200 emails written in chineese among which 100 are spam and 100 non-spam. Do you think someone who has never had any training about chineese language would be able to reach the kind of generalization accuracy a computer would?
The examples above illustrate how little information computers have when they are faced with a supervised learning task. It seems reasonable to assume that humans faced with similar tasks would not be much better.
The above discussion aims at emphasizing the importance of "having the right prior". To some extent, building an algorithm essentially means designing a prior, and designing a prior can only be done with respect to a class of problems (there is no "universal" prior). Designing a prior (which also means choosing an appropriate representation of the data) actually allows to introduce a large amount of information into the learning algorithm. Most human learning tasks are tasks that require a lot of information and this is why computers usually fail on those.
GOD will lead the way. Keep the faith, hope and courage. Believe in miracle. There is no impossible to GOD. "Put GOD on top of everything you do and you wouldn't go wrong because in GOD we trust and nothing hard at all"
About Me
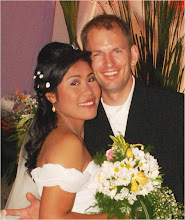
- Eugielene (Ladaga) Tulin Nonweiler
- I am born not rich but rich in love. I have a good family, good friends. I already taste the life's bitterness but also taste life's sweetness. I am just simple, Friendly and understanding. GOD fearing. I believe in GOD so much. I just cant explain it here but deep in my heart I know , I feel it.
Wednesday, November 23, 2005
Wednesday, November 16, 2005
Thursday, November 03, 2005
The Halloween
Halloween is one of another busy days here in our place. During this time, we go to our granparents house. This is really what we did eveytime when this holiday come. Its a part of our culture that we will all went to our grandparents house. We did fun chats. And we watched movies.
During the night, we all agreed to watched a horror movie. Then there goes, we all break into hestirical shout... because everytime the ghost show in the screen it makes our skin feel cold like someone holding us. And we also feel that somewhere in the dark place there they are wating for us..... grrrrrrrr......... After the movie, we did some talk and chating about more horror movies. After that we all, its time to sleep but.... the problem is we all scared to sleep alone in our room. So, what we did was we all sleep in one room. Though it make us fit so much... And until out room, we still talking to what we have seen in the movie.... I think I fell asleep already past midnight........
Happy Halloween to all....
EUGIE
Yahoo! FareChase - Search multiple travel sites in one click.
Subscribe to:
Posts (Atom)